Abstract
In the previous two posts, we examined the Actiwatch 2 algorithm for deriving sleep/wake patterns and sleep parameters from raw acceleration (ACC) data. This post applied the same algorithms to 10 nights of raw-ACC data obtained from Vivosmart 4 and compared the results with Actiwatch 2.
As described here, we found both the sleep/wake patterns and sleep parameters derived from Vivosmart 4 to be consistent with that of Actiwatch 2. The result suggests that an affordable, consumer wearable such as Vivosmart 4 can reproduce the results from a more expensive, medical-grade actigraphic device like Actiwatch 2.
1. Comparison of Sleep/Wake Detection and Sleep Parameters
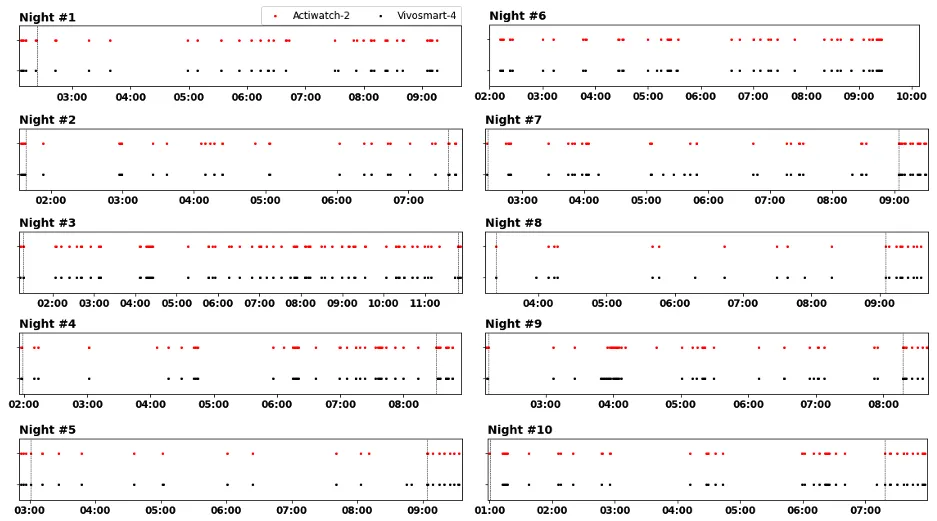
Figure 1 illustrates the sleep/wake patterns of the 10 nights derived from Actiwatch 2 and Vivosmart 4 (the method was introduced in a previous post). The two vertical dashed lines in each subplot indicated the Actiwatch 2 scored sleep onset and offset. Table 1 lists the consistent and inconsistent results between Actiwatch 2 and Vivosmart 4. The number in each cell is the total minutes within sleep onset and offset in the 10 nights. Treating the result from Actiwatch 2 as a standard, the sensitivity (to detect sleep), specificity, and accuracy of Vivosmart 4 are near 1.00, 0.84, and 0.99, respectively.
.webp)
Table 2 compares the differences in sleep parameters (introduced in this article) between Actiwatch 2 and Vivosmart 4. The two watches showed good consistency as the mean differences of most of the parameters is smaller than 5 mins.

2. Conclusion
We examined the full algorithm utilized in the Actiwatch 2 to derive nighttime sleep/wake patterns and sleep parameters from the raw acceleration signal. Both the sleep/wake patterns and sleep parameters derived from Vivosmart 4 are consistent with that of Actiwatch 2. The result suggests that an affordable, consumer wearable such as Vivosmart 4 can reproduce the results from a more expensive, medical-grade actigraphic device.
3. References
[1] K. Y. Chen and D. R. Bassett, “The technology of accelerometry-based activity monitors: current and future,” Med. Sci. Sports Exer., vol. 37, pp. S490–S500, Nov. 2005.
[2] CamNtech, “The MotionWatch 8 and MotionWare User Guide.”
[3] Respironics, “Instruction manual of Actiwatch Communication and Sleep Analysis Software. “
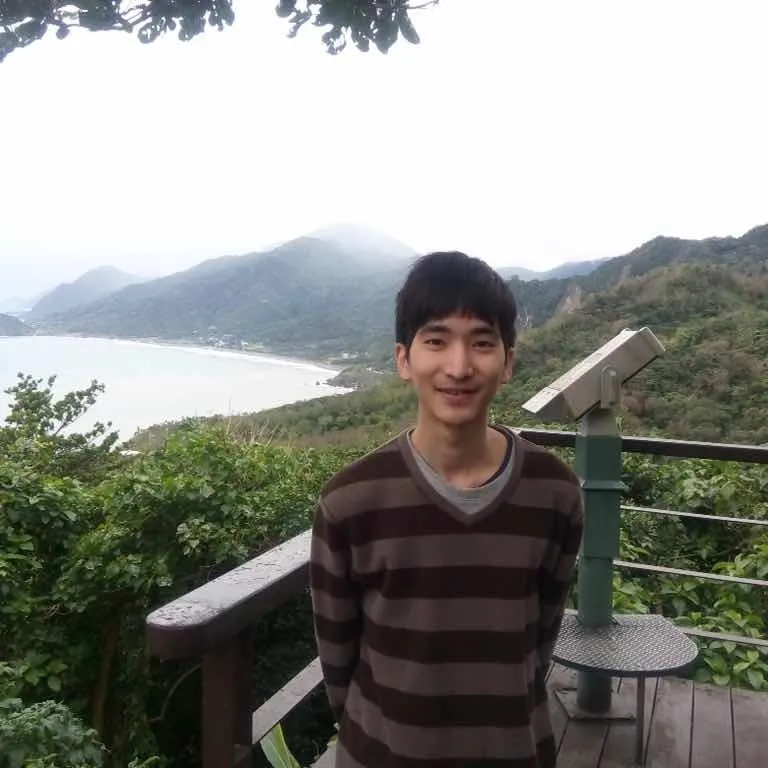
Francis is a research Lead at Labfront, responsible for data validation and analysis. He is interested in applying physics or math to medical research.
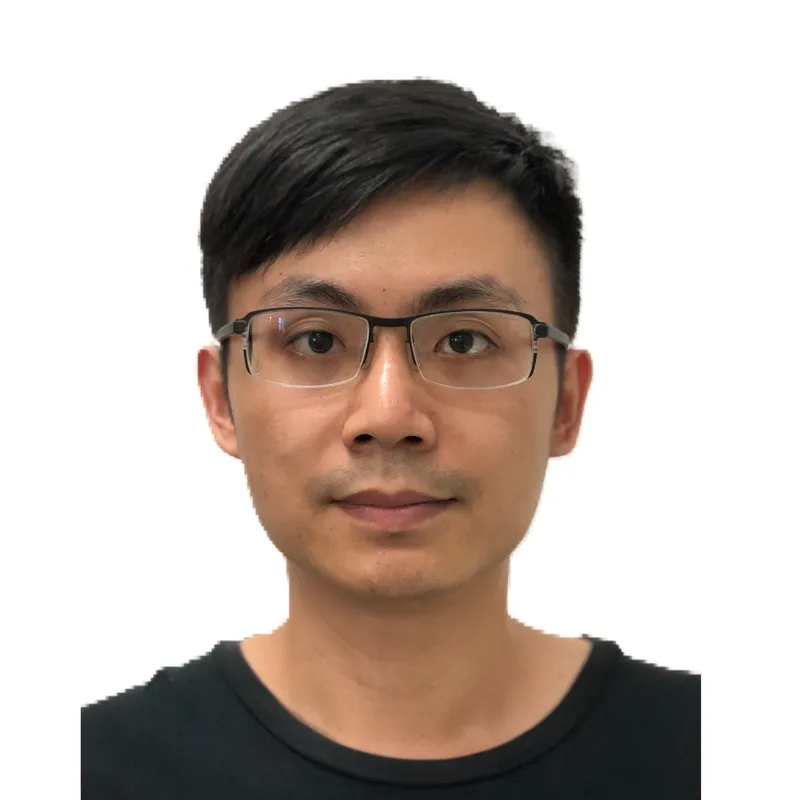
Han-Ping is the senior research lead (and chief plant caretaker) at Labfront, specializing in physiological data analysis. An alumnus of the BIDMC/Harvard's Center for Dynamical Biomarkers, Han-Ping uses his PhD in electrophysics to help Labfront customers convert raw physiological data into health insights. He does his best Python coding while powered by arm massages from his spiky-tongued cat, Pi.
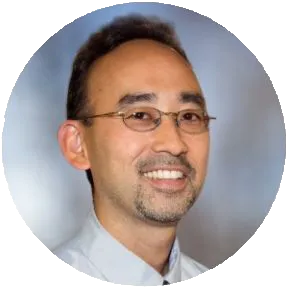
Dr. Ahn is an internal medicine physician with a background in physics/engineering and physiological signal analyses. He is the Chief Medical Officer at Labfront and an Assistant Professor in Medicine & Radiology at Harvard Medical School. Dr. Ahn is passionate about democratizing health sciences and exploring health from an anti-disciplinary perspective.