Abstract
Following our previous post on deriving sleep/wake patterns from the acceleration data of Motionlogger, this article gives a brief demonstration of how the various sleep parameters are derived according to a detailed-written paper proposed by Fekedulegn et al. [1].
1. Deriving Sleep Parameters from Sleep/Wake Patterns
Figure 1 shows the sleep/wake patterns derived from Motionlogger, as introduced in a previous post. Some important sleep events are labeled, such as bedtime, get-up time, sleep onset, sleep offset, and wake episodes. Motionlogger has an event marker button for users to record when they go to bed (Bed Time) and get out of bed (Get Up Time). Sleep onset is the starting time point of the first continuous block of at least 20 min of sleep with no more than 1 min of interruption and sleep offset as the last minute the subject was scored asleep before getting out of bed [1].
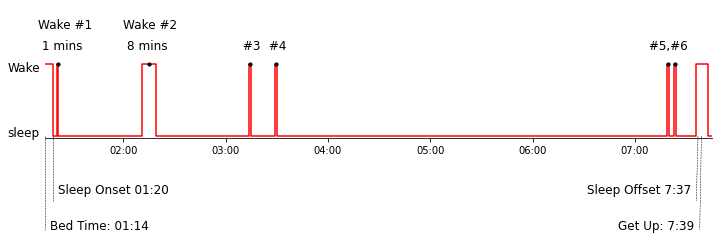
Table 1 then illustrates the resulting 7 sleep parameters, which are usually adopted in a sleep report.
In addition to these 7 commonly used parameters, Fekedulegn et al. introduced another 22 parameters with detailed algorithms with examples in an Excel file [1].
.png)
2. References
[1] D. Fekedulegn, M. E. Andrew, M. Shi, J. M. Violanti, S. Knox, and K. E. Innes, "Actigraphy-based assessment of sleep parameters," Annals of Work Exposures and Health, vol. 64, no. 4, pp. 350-367, 2020.
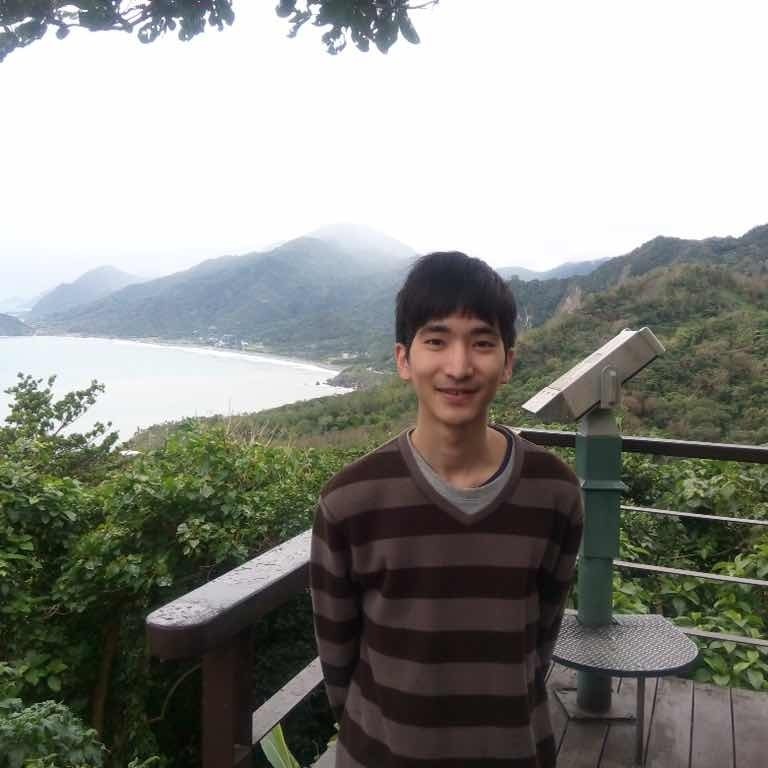
Francis is a research Lead at Labfront, responsible for data validation and analysis. He is interested in applying physics or math to medical research.

Han-Ping is the senior research lead (and chief plant caretaker) at Labfront, specializing in physiological data analysis. An alumnus of the BIDMC/Harvard's Center for Dynamical Biomarkers, Han-Ping uses his PhD in electrophysics to help Labfront customers convert raw physiological data into health insights. He does his best Python coding while powered by arm massages from his spiky-tongued cat, Pi.
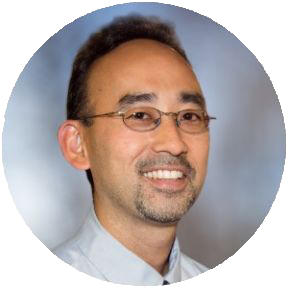
Dr. Ahn is an internal medicine physician with a background in physics/engineering and physiological signal analyses. He is the Chief Medical Officer at Labfront and an Assistant Professor in Medicine & Radiology at Harvard Medical School. Dr. Ahn is passionate about democratizing health sciences and exploring health from an anti-disciplinary perspective.